Machine Vision System for Printing Manufacturers
Highlights
In 2020, we were contacted by a full-cycle flexo printing house – a leading manufacturer of flexible packaging and self-adhesive labels. To cut the film, the client uses a slitter machine that operates at very high speeds. The client used to miss up to 90% of film defects, since they were reliant on a person working as a spotter and the high operating speeds made errors difficult to catch.
Challenge
Customer Challenge
Eliminate the human factor in film defect detection to prevent the shipping of defective products to end customers and losing money through complaints.
Project Challenge
Develop a cost-effective defect tracking solution for use in production, including alarms, to substantially reduce the volume of defects by excluding the human factor in the detection process
Solution
To ensure top quality of the film cut at very high speeds, the PSA team designed the system to automatically detect printing gaps and signal to stop the slitter machine. We utilized machine vision technology within the system and reduced technical requirements for the camera by utilizing a neural network. It analyzes the captured images of film and is trainable to detect printing gaps.
To ensure the effective operation of the machine vision system, we provided the following features:
-
Capturing and processing video information from a film moving at a speed of 50 to 1000 meters per minute
-
Light and sound signaling indication
-
Speed and contrast measurements via external sensors
The architecture of the integrated hardware and software solution is shown in the picture below. For the rapid development and debugging of an industrial prototype of a system, we utilized the Toradex Colibri module that features a single-core CPU with 800 MHz clock speed, which allows for recognition of images at high speeds. To complete the customization we used the Yocto Project for integrating the custom layers into the build.
For all the components to work seamlessly within a system, we provided:
-
Communication with a server to be informed about the actual status of slitter machines and the detection of any unprinted samples
-
Transmission of the video stream to the neural network for gap detection
-
External microcontroller to measure the tape speed and provide crypto protection
-
Web interface for operators to interact with the system
We ensured robust communication for all components, as well as implemented the licensing service. To identify if a section of the tape was printed well, we used the video stream broadcast service that was based on a package distributed with the Yocto Project — mjpg_streamer. Thus, when a gap is detected, the operator gets the signal to stop the machine immediately.
The delivered device is subject to intellectual property rights, equipped with speed sensors, and a camera located above the printed area, which is connected to the server app that transmits the video stream to the neural network for its analysis.
Development included
- Embedded Software Development
- Server Software Development
- Web Interface Development
- Hardware Development
- System Electronics Design
Result
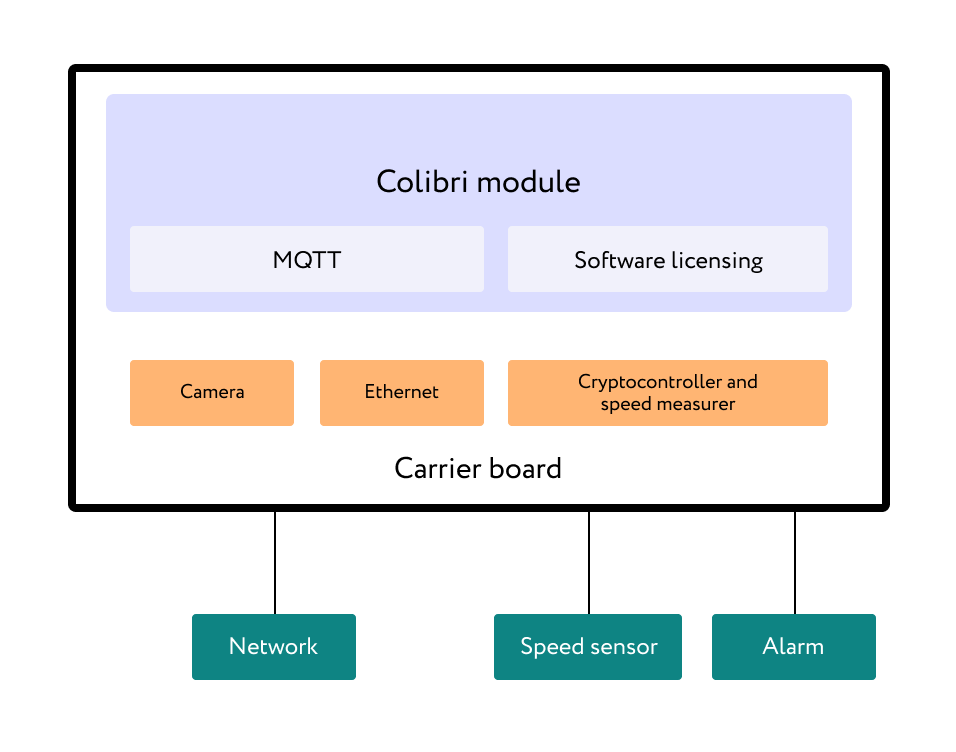
-
Implemented solution successfully in production 2 weeks ahead of expected deadline
-
30% cost reduction over alternative solution
-
Number of defects reduced by 90%
-
ROI is 1 year
Technology Breakdown
- 1 Senior Software Engineer
- 2 Software Engineers
- 2 Technical Coordinators
- 1 Project Manager
- 1 Technical Assistant
- 1 Designer
- From December 2020 to February 2022